Example 2: Synthetic Data Fit#
In this example, we compare the DRD model to the Mann model, using the three IEC-recommended Mann model parameters: \(L/\text{zref}=0.59, Γ=3.9, αϵ^{2/3}=3.2 * (\text{zref}^{2/3} / \text{ustar}^2)\). In this example, the exponent \(\nu=-\frac{1}{3}\) is fixed so that \(\tau(\boldsymbol{k})\) matches the slope of \(\tau^{IEC}\) for in the energy-containing range, \(k \rightarrow 0\).
The following example is also discussed in the original DRD paper.
Import packages#
First, we import the packages we need for this example. Additionally, we choose to use CUDA if it is available.
[1]:
import torch
import torch.nn as nn
from drdmannturb import EddyLifetimeType
from drdmannturb.parameters import (
LossParameters,
NNParameters,
PhysicalParameters,
ProblemParameters,
)
from drdmannturb.spectra_fitting import CalibrationProblem, OnePointSpectraDataGenerator
device = "cuda" if torch.cuda.is_available() else "cpu"
if torch.cuda.is_available():
torch.set_default_tensor_type("torch.cuda.FloatTensor")
Setting Physical Parameters#
The following cell sets the necessary physical parameters
\(L\) is our characteristic length scale, \(\Gamma\) is our characteristic time scale, and \(\sigma = \alpha\epsilon^{2/3}\) is the spectrum amplitude.
[2]:
zref = 90 # reference height
z0 = 0.02
zref = 90
uref = 11.4
ustar = 0.556 # friction velocity
# Scales associated with Kaimal spectrum
L = 0.593 * zref # length scale
Gamma = 3.89 # time scale
sigma = 3.2 * ustar**2.0 / zref ** (2.0 / 3.0) # magnitude (σ = αϵ^{2/3})
print(f"Physical Parameters: {L,Gamma,sigma}")
k1 = torch.logspace(-1, 2, 20) / zref
Physical Parameters: (53.37, 3.89, 0.04925737023046032)
CalibrationProblem
construction#
We’ll use a simple neural network consisting of two layers with \(10\) neurons each, connected by a ReLU activation function. The parameters determining the network architecture can conveniently be set through the NNParameters
dataclass.
Using the ProblemParameters
dataclass, we indicate the eddy lifetime function \(\tau\) substitution, that we do not intend to learn the exponent \(\nu\), and that we would like to train for 10 epochs, or until the tolerance tol
loss (0.001 by default), whichever is reached first.
Having set our physical parameters above, we need only pass these to the PhysicalParameters
dataclass just as is done below.
Lastly, using the LossParameters
dataclass, we introduce a second-order derivative penalty term with weight \(\alpha_2 = 1\) and a network parameter regularization term with weight \(\beta=10^{-5}\) to our MSE loss function.
[3]:
pb = CalibrationProblem(
nn_params=NNParameters(
nlayers=2,
# Specifying the hidden layer sizes is done by passing a list of integers, as seen here.
hidden_layer_sizes=[10, 10],
# Specifying the activations is done similarly.
activations=[nn.ReLU(), nn.ReLU()],
),
prob_params=ProblemParameters(
nepochs=10, learn_nu=False, eddy_lifetime=EddyLifetimeType.TAUNET
),
# Note that we have not activated the first order term, but this can be done by passing a value for ``alpha_pen1``
loss_params=LossParameters(alpha_pen2=1.0, beta_reg=1.0e-5),
phys_params=PhysicalParameters(
L=L, Gamma=Gamma, sigma=sigma, ustar=ustar, domain=k1
),
logging_directory="runs/synthetic_fit",
device=device,
)
Data Generation#
We now collect Data = (<data points>, <data values>)
and specify the reference height (zref
) to be used during calibration. Note that DataType.KAIMAL
is used by default.
[4]:
Data = OnePointSpectraDataGenerator(data_points=k1, zref=zref, ustar=ustar).Data
Calibration#
Now, we fit our model. CalibrationProblem.calibrate
takes the tuple Data
which we just constructed and performs a typical training loop.
[5]:
optimal_parameters = pb.calibrate(data=Data)
pb.print_calibrated_params()
========================================
Initial loss: 0.04606855911763526
========================================
100%|████████████████████████████████████████████████████████████████| 10/10 [00:21<00:00, 2.19s/it]
========================================
Spectra fitting concluded with final loss: 0.008501029301051776
========================================
Optimal calibrated L : 54.5565
Optimal calibrated Γ : 1.4951
Optimal calibrated αϵ^{2/3} : 0.0460
========================================
Plotting#
DRDMannTurb
offers built-in plotting utilities and Tensorboard integration which make visualizing results and various aspects of training performance very simple.
The following will plot the fit.
[6]:
pb.plot()
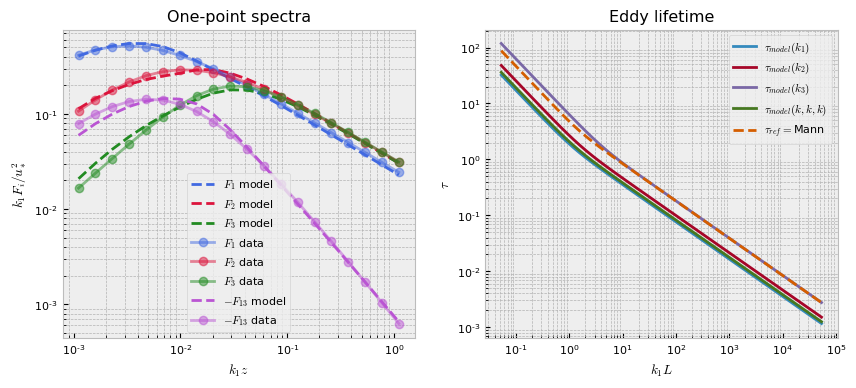
This plots out the loss function terms as specified, each multiplied by the respective coefficient hyperparameter. The training logs can be accessed from the logging directory with Tensorboard utilities, but we also provide a simple internal utility for a single training log plot.
[7]:
pb.plot_losses(run_number=0)
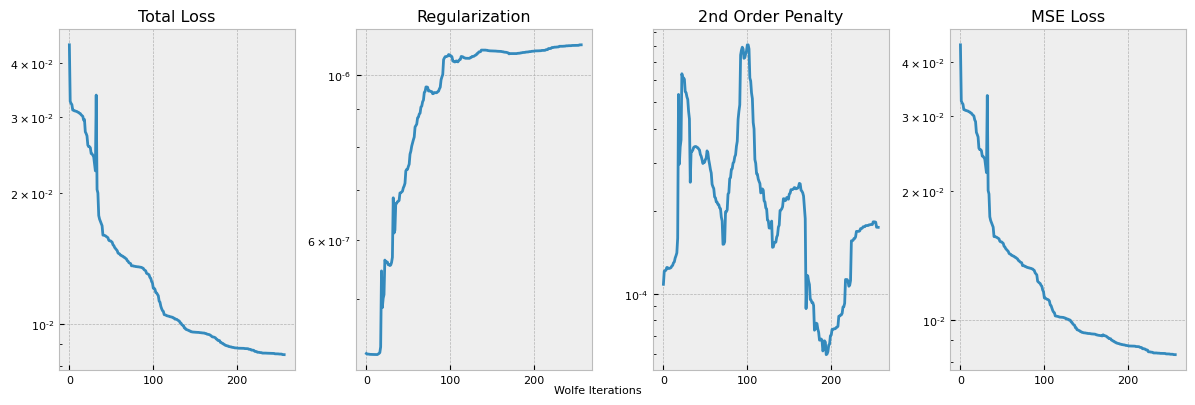
Save Model with Problem Metadata#
Here, we’ll make use of the model saving utilities, which make saving the DRDMannTurb
fit very straightforward. The following line automatically pickles and writes out a trained model along with the various parameter dataclasses in ../results
.
[8]:
pb.save_model("./outputs/")
Loading Model and Problem Metadata#
Lastly, we load our model back in.
[9]:
import pickle
path_to_parameters = "./outputs/EddyLifetimeType.TAUNET_DataType.KAIMAL.pkl"
with open(path_to_parameters, "rb") as file:
(
nn_params,
prob_params,
loss_params,
phys_params,
model_params,
) = pickle.load(file)
Recovering Old Model Configuration and Old Parameters#
We can also load the old model configuration from file and create a new CalibrationProblem
object from the stored network parameters and metadata.
[10]:
pb_new = CalibrationProblem(
nn_params=nn_params,
prob_params=prob_params,
loss_params=loss_params,
phys_params=phys_params,
device=device,
)
pb_new.parameters = model_params
import numpy as np
assert np.ma.allequal(pb.parameters, pb_new.parameters)